Palm Oil Price Forecasting: Techniques and Trends
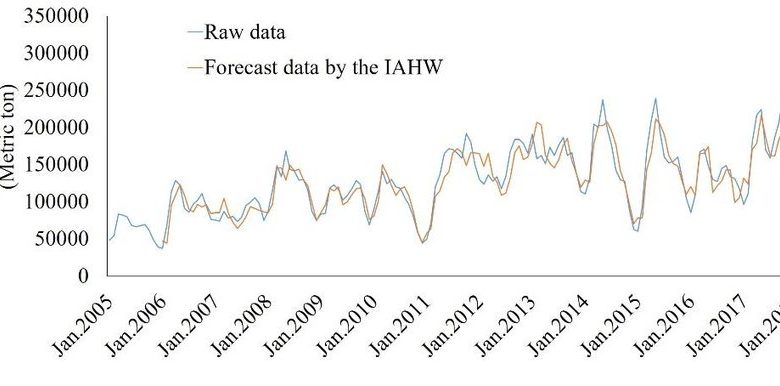
Accurate palm oil price forecasting is essential for stakeholders in the palm oil industry, including producers, traders, investors, and policymakers. Palm oil prices are influenced by a complex interplay of factors such as production levels, weather conditions, geopolitical events, and market demand.
Reliable forecasts help stakeholders make informed decisions about production planning, inventory management, trading strategies, and risk mitigation. By anticipating price movements, stakeholders can optimize their operations, enhance profitability, and navigate market uncertainties more effectively.
Forecasting Techniques: Overview of Methods
1. Statistical Analysis
Statistical analysis involves using historical price data and identifying patterns and trends to predict future prices. Techniques such as moving averages, autoregressive integrated moving average (ARIMA) models, and seasonal decomposition of time series data (STL) are commonly used. These methods help in understanding the underlying price dynamics and forecasting short-term price movements.
Moving Averages: This method smooths out price data to identify trends by averaging prices over a specified period. Simple moving averages (SMA) and exponential moving averages (EMA) are popular variants.
ARIMA Models: ARIMA models are used to analyze and forecast time series data by accounting for autocorrelation and differencing. These models are effective in capturing linear relationships in the data.
Seasonal Decomposition: STL decomposes time series data into trend, seasonal, and residual components, providing insights into seasonal patterns and long-term trends.
2. Econometric Models
Econometric models incorporate economic theories and relationships between variables to forecast prices. These models consider factors such as supply and demand dynamics, exchange rates, and macroeconomic indicators.
Common econometric techniques include multiple regression analysis, vector autoregression (VAR), and cointegration analysis.
Multiple Regression Analysis: This technique models the relationship between palm oil prices and multiple explanatory variables, such as production levels, import/export data, and economic indicators.
Vector Autoregression (VAR): VAR models capture the interdependencies between multiple time series variables, allowing for the analysis of how changes in one variable affect others over time.
Cointegration Analysis: This method identifies long-term equilibrium relationships between variables, helping to forecast price movements based on underlying economic principles.
3. Machine Learning
Machine learning (ML) techniques have gained prominence in price forecasting due to their ability to handle large datasets and complex nonlinear relationships. Algorithms such as neural networks, support vector machines (SVM), and random forests are commonly used.
Neural Networks: Neural networks, particularly recurrent neural networks (RNN) and long short-term memory (LSTM) networks, are effective in capturing temporal dependencies and patterns in time series data.
Support Vector Machines (SVM): SVMs are used for regression and classification tasks, providing robust predictions by identifying optimal hyperplanes that separate data points.
Random Forests: This ensemble learning method combines multiple decision trees to improve predictive accuracy and handle high-dimensional data.
Case Studies: Examples of Successful and Failed Forecasts in the Palm Oil Market
1. Successful Forecasts
2016 Price Surge: Analysts successfully predicted the price surge in 2016 due to El Niño-induced droughts in Southeast Asia, which significantly reduced palm oil production. Forecasts based on weather patterns and historical data helped stakeholders prepare for the price increase.
Post-2020 Recovery: Following the COVID-19 pandemic, analysts anticipated a recovery in palm oil prices due to increased demand from the food and biofuel industries.
Machine learning models incorporating economic recovery indicators and trade data provided accurate forecasts, aiding market participants in strategic decision-making.
2. Failed Forecasts
2008 Financial Crisis: The global financial crisis led to unexpected price volatility in commodities, including palm oil. Traditional econometric models failed to predict the extent of the price collapse, highlighting the limitations of relying solely on historical data without considering broader economic disruptions.
2019 Trade War Impact: The US-China trade war caused unforeseen fluctuations in palm oil prices. Many forecasts did not fully account for the rapid changes in trade policies and their ripple effects on global commodity markets, leading to inaccuracies in price predictions.
Emerging Trends: New Developments in Forecasting Technology and Practices
1. Integration of Big Data and IoT
The integration of big data and the Internet of Things (IoT) is revolutionizing price forecasting. IoT devices provide real-time data on weather conditions, soil moisture levels, and crop health, enabling more accurate and timely predictions.
Big data analytics allows for the processing and analysis of vast amounts of data from various sources, enhancing the precision of forecasts.
2. Advanced Machine Learning Techniques
Advanced machine learning techniques, such as deep learning and ensemble methods, are improving the accuracy of price forecasts. These techniques can capture complex, nonlinear relationships in the data and adapt to changing market conditions.
For example, ensemble methods like stacking and boosting combine multiple models to reduce prediction errors and improve robustness.
3. Scenario Analysis and Stress Testing
Scenario analysis and stress testing are becoming integral to price forecasting practices. These methods involve simulating different scenarios, such as geopolitical events, economic shocks, and environmental changes, to assess their potential impact on palm oil prices.
This approach helps stakeholders prepare for various contingencies and enhances the resilience of their strategies.
4. Collaborative Forecasting Platforms
Collaborative forecasting platforms enable stakeholders to share data, insights, and models, fostering a more comprehensive and accurate forecasting process. These platforms leverage collective intelligence and diverse expertise, improving the reliability of predictions and facilitating better decision-making.
Conclusion
Accurate palm oil price forecasting is crucial for stakeholders in navigating the complexities of the global market. Various techniques, including statistical analysis, econometric models, and machine learning, offer valuable tools for predicting price movements.
Successful and failed forecasts highlight the importance of considering multiple factors and adapting to changing conditions. Emerging trends, such as the integration of big data, advanced machine learning, scenario analysis, and collaborative platforms, are enhancing the precision and reliability of price forecasts.
By leveraging these advancements, stakeholders can optimize their strategies, manage risks, and capitalize on market opportunities.